Enhancing Business Efficiency with Machine Learning Data Labeling
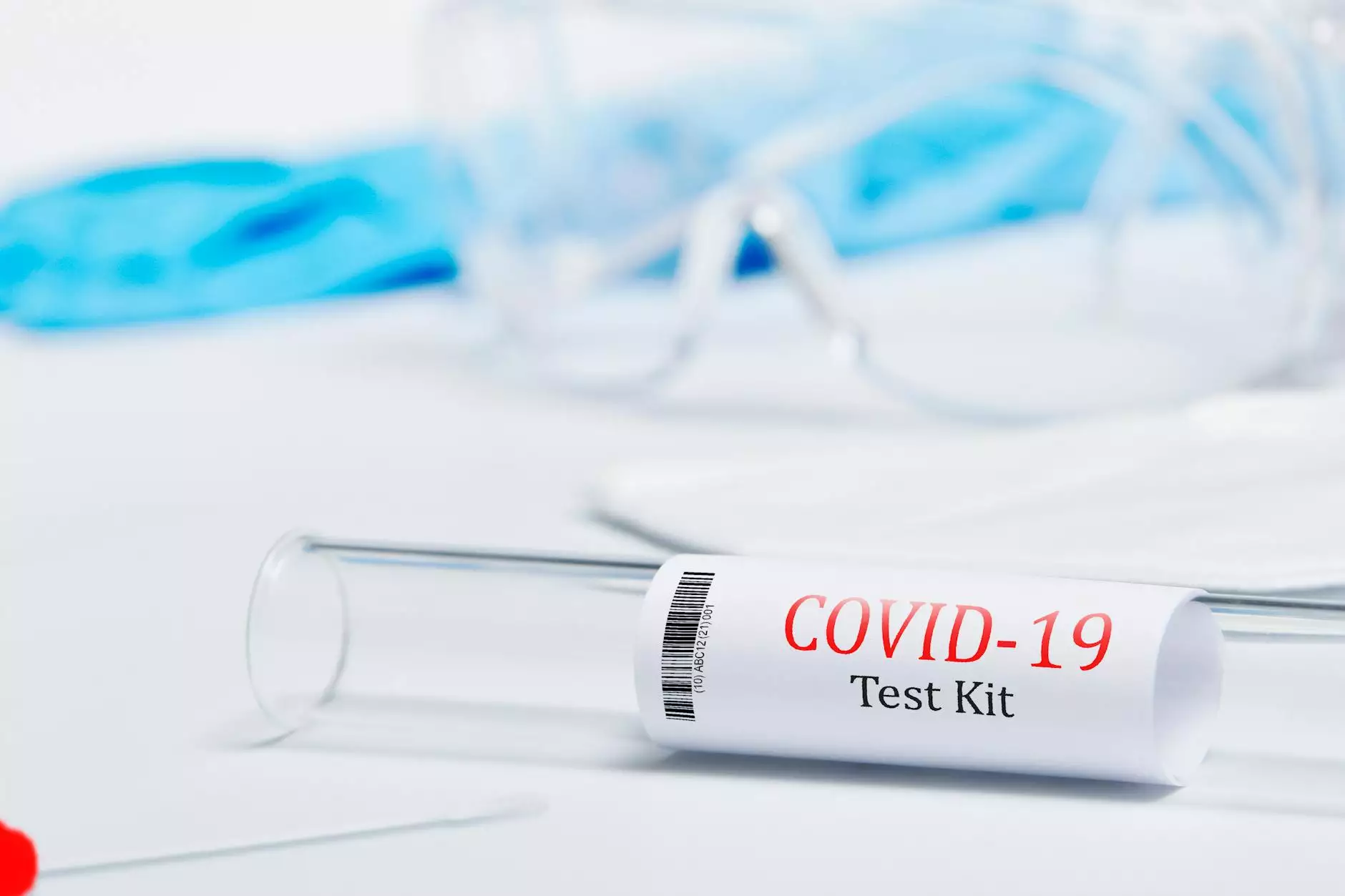
Machine learning data labeling has become an integral part of modern business strategies, especially in sectors such as home services and locksmith operations. This technique not only aids in the accurate training of machine learning models but also enhances operational efficiency and customer satisfaction. In this article, we will delve into the various aspects of machine learning data labeling and how businesses can utilize it to stay competitive.
The Significance of Machine Learning Data Labeling
Data labeling is the process of annotating or tagging data, which is crucial for supervised machine learning. Here, data scientists provide algorithms with labeled datasets so that the models can learn to make predictions or classifications accurately.
1. Improving Accuracy in Business Predictions
One of the primary benefits of machine learning data labeling is the improvement in accuracy for various predictive models. In business contexts, this can range from predicting customer behavior to optimizing inventory management. Accurate predictions lead to:
- Increased Sales: By understanding customer preferences, businesses can tailor their products and marketing strategies accordingly.
- Reduced Costs: Better forecasting prevents overproduction and stock shortages.
2. Personalizing Customer Experiences
With the help of labeled data, businesses can harness machine learning to deliver personalized experiences to their customers. For example, locksmiths can utilize previous customer interactions to suggest relevant services or products during subsequent engagements.
3. Enhancing Operational Efficiency
By integrating machine learning data labeling into their operations, businesses can automate various processes, thus reducing the time and resources spent on tasks that can be efficiently performed by machine learning models. Some areas where efficiency can be improved include:
- Dispatching Services: Optimizing route and time for service calls, particularly for locksmiths.
- Inventory Management: Tracking stock levels more effectively through predictive analysis.
Applications of Machine Learning Data Labeling in Home Services and Locksmiths
1. Predictive Maintenance
In the home services industry, predictive maintenance is essential. By labeling data from previous service records, businesses can predict when a machine or appliance is likely to fail, allowing proactive maintenance before problems arise.
2. Intelligent Dispatching for Locksmith Services
Locksmiths can utilize machine learning to determine the best technician to respond to a service call based on various factors such as location, expertise, and current workload. Properly labeled data regarding technician performance can assist in making this determination.
3. Fraud Detection
Using labeled data, businesses can employ machine learning algorithms to identify patterns associated with fraudulent activities. This can be particularly useful in locksmith services where unauthorized services may be proposed.
Implementing Machine Learning Data Labeling
To successfully implement machine learning data labeling, consider the following steps:
1. Identify Data Sources
Begin by identifying all possible data sources related to your business operations. This might include customer feedback, service records, and inventory databases.
2. Label Your Data Accurately
Engage skilled annotators or use automated tools to ensure your data is accurately labeled. This step is crucial as the quality of labeled data directly impacts the performance of machine learning models.
3. Train Your Models
After successful labeling, divide your data into training and testing datasets. Use the training data to teach your machine learning model and the testing data to evaluate its performance.
4. Continuous Improvement
Machine learning models require continuous monitoring and updating. Regularly retrain your models with new labeled data to ensure they remain accurate over time.
Challenges in Machine Learning Data Labeling
While the benefits are significant, businesses may also face challenges in implementing machine learning data labeling. These include:
1. Resource Intensive
Data labeling can be resource-intensive, requiring time and financial investment, especially if the data volume is large.
2. Expertise Requirement
Labels must be accurate to be effective, which may necessitate hiring skilled data scientists or annotators familiar with the specific business context.
3. Maintaining Data Privacy
When handling customer data, businesses must ensure compliance with data protection regulations. Proper anonymization and secure handling of sensitive information are paramount.
Future Trends in Machine Learning Data Labeling
As technology continues to evolve, the techniques and applications of machine learning data labeling will diversify. Here are some trends to watch:
1. Automation of Data Labeling
With advancements in artificial intelligence, automated data labeling tools are becoming more prevalent, reducing the need for manual intervention.
2. Integration with Other Technologies
Machine learning will increasingly be integrated with other technologies, such as the Internet of Things (IoT) and blockchain, to enhance transparency and efficiency in operations.
3. Enhanced Data Ethics
As businesses become more data-driven, ethical concerns around data privacy and usage will lead to stricter regulations and standards for data labeling practices.
Conclusion
In conclusion, machine learning data labeling is vital for businesses looking to improve their operations, particularly in the competitive home services and locksmith industries. By accurately labeling data, companies can reap the benefits of enhanced accuracy, personalized services, and improved operational efficiencies. As the field of machine learning evolves, businesses that effectively implement these strategies will likely see significant advantages in their market performance.
For those interested in staying ahead of the curve, understanding and utilizing machine learning data labeling is not just an option; it is a necessity for growth and innovation in today’s business landscape.