Data Annotation for Machine Learning: Empowering Home Services and Locksmiths
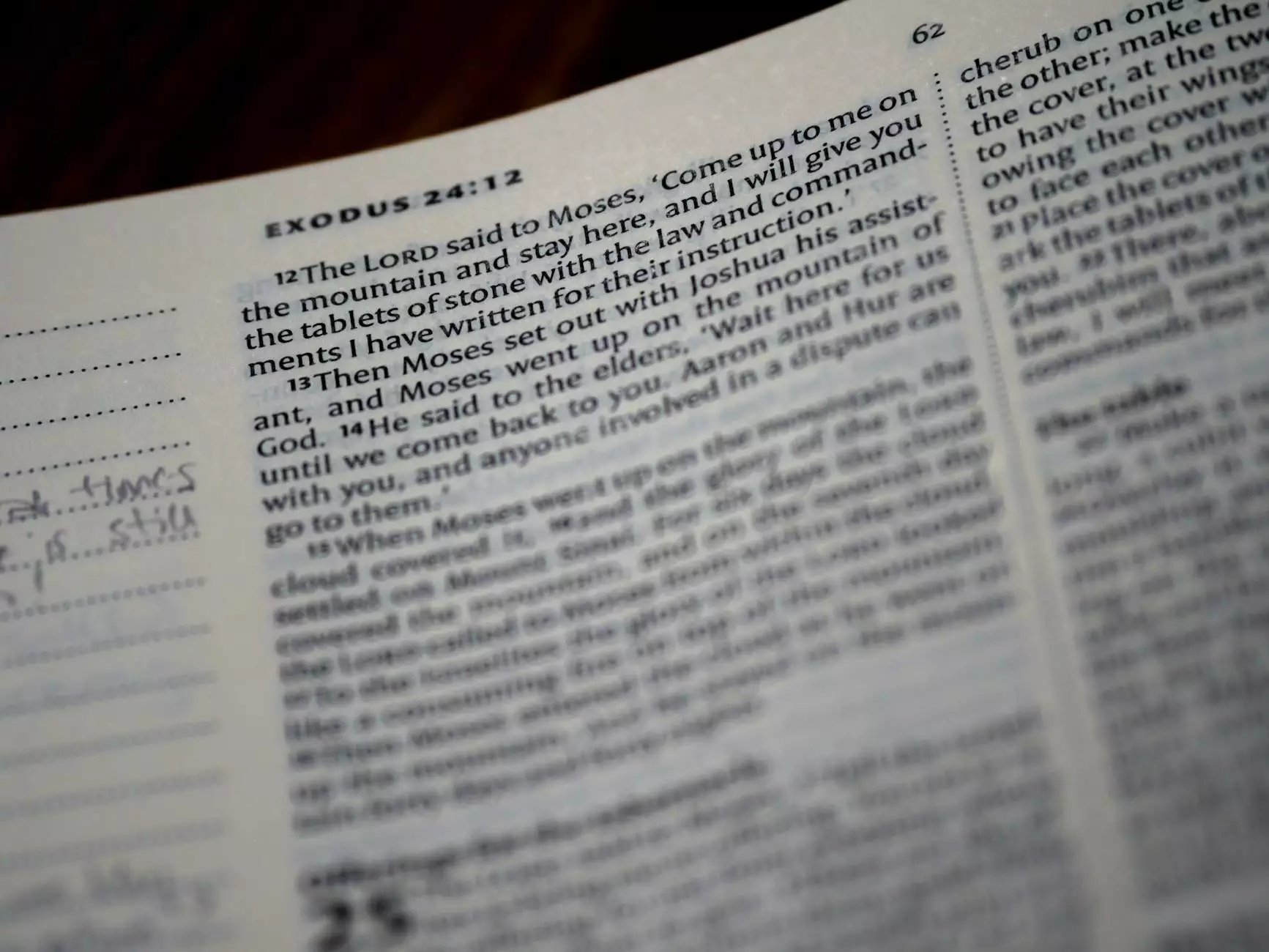
In today’s fast-paced digital landscape, businesses in the Home Services and Keys & Locksmiths sectors are leveraging innovative technologies to enhance service delivery and customer satisfaction. One of the most transformative technologies in recent years is data annotation for machine learning. By ensuring that data is accurately labeled and categorized, businesses can develop advanced machine learning algorithms that improve their operational efficiency, optimize service offerings, and ultimately provide better customer experiences.
Understanding Data Annotation in Machine Learning
Data annotation involves the process of labeling data, enabling machine learning algorithms to learn from it. This is a crucial step in the machine learning lifecycle, as the quality of annotated data directly impacts the performance of the model. Simply put, without accurate data annotation, machine learning systems can misinterpret data, leading to poor decision-making and ineffectual results.
The Importance of Data Annotation for Businesses
For businesses in the locksmith and home services industry, the benefits of employing data annotation machine learning strategies are profound:
- Improved Decision-Making: Annotated data allows businesses to make informed decisions by analyzing patterns and trends.
- Enhanced Customer Insights: Understanding customer behavior through properly annotated data can help tailor services to meet client needs.
- Operational Efficiency: Machine learning models can automate repetitive tasks, thus improving efficiency and reducing human error.
- Competitive Advantage: Leveraging data annotation empowers businesses to stay ahead of the competition by applying advanced analytics.
The Data Annotation Process Explained
The data annotation process can be broken down into several key steps that are vital for effective implementation:
1. Data Collection
The first step involves gathering relevant data, which could be images, text, audio, or videos depending on the services provided. For locksmiths, this might include images of locks, customer requests, or even recorded calls for service.
2. Data Preprocessing
Once the data is collected, it needs to be cleaned and organized. This involves removing any noise or irrelevant information that could skew results in the machine learning processes.
3. Annotation by Experts
Data must be accurately labeled, often requiring human oversight. For example, an expert might categorize different types of locks or classify customer inquiries based on urgency.
4. Quality Assurance
After labeling, quality checks are essential to ensure the annotations are precise and reliable. This step safeguards the integrity of the training dataset.
5. Training Machine Learning Models
With annotated data, businesses can now train their machine learning models, helping them understand and process information effectively, thus improving predictive capabilities.
How Data Annotation Impacts the Locksmith Industry
The locksmith industry is evolving due to advancements in technology and customer expectations. Implementing data annotation machine learning can revolutionize various facets of this industry:
Automating Customer Service
By utilizing chatbots trained on annotated data, locksmith services can automate common queries. For instance, chatbots can handle bookings, respond to pricing inquiries, and provide basic troubleshooting tips for customers in need of emergency services. This not only improves customer service but also reduces the workload on human agents, allowing them to focus on more complex issues.
Predictive Maintenance and Service Optimization
With machine learning models trained on historical service data, locksmith businesses can predict when a lock might need maintenance or replacement. This proactive approach can prevent emergency situations and enhance customer satisfaction.
Enhanced Marketing Strategies
Analyzing customer data allows locksmith businesses to tailor their marketing strategies effectively. By understanding demographics and customer preferences through annotated data, these businesses can create targeted marketing campaigns that resonate with their audience, thus increasing conversion rates.
The Role of Technology in Data Annotation
Modern technologies play a significant role in enhancing the data annotation process. Here are some key technologies shaping the future of data annotation for machine learning:
1. AI-Powered Annotation Tools
AI-driven tools can significantly speed up the data annotation process by automating parts of the task. These tools rapidly analyze large datasets, identify patterns, and suggest annotative categories, reducing the time taken for human intervention.
2. Crowdsourcing Platforms
Businesses can utilize crowdsourcing platforms to expand their pool of annotators, thus facilitating faster data labeling at reduced costs. This is particularly effective when vast amounts of data need to be annotated quickly.
3. Cloud-Based Solutions
Utilizing cloud-based data annotation solutions allows for seamless collaboration among teams. Annotators from different locations can access data and contribute to the project in real time, ensuring a collaborative approach to data labeling.
Challenges in Data Annotation for Machine Learning
Despite the numerous advantages of data annotation machine learning, businesses may encounter several challenges:
1. Data Privacy Concerns
Handling sensitive customer data, especially in the locksmith industry, raises privacy issues. It's imperative for companies to comply with data protection regulations and ensure that customer data is secure during the annotation process.
2. Cost Implications
Annotating data is often resource-intensive. Companies must consider the costs of hiring skilled annotators or investing in advanced annotation tools to ensure accurate labeling.
3. Ensuring Consistency and Accuracy
Maintaining consistency across annotations can be challenging, especially with numerous annotators. Establishing clear guidelines and conducting regular reviews can mitigate discrepancies.
Future of Data Annotation in Home Services
As technology continues to evolve, the future of data annotation for machine learning in the home services and locksmith industry looks promising:
1. Increasing Dependence on Machine Learning
More businesses will rely on machine learning to enhance their operational efficiency and customer service. As demands grow, so will the need for high-quality annotated data.
2. Enhanced AI Capabilities
With advancements in AI, we can expect improved annotation tools that require less human oversight, further streamlining the data annotation process.
3. Integration with IoT and Smart Locks
The rise of IoT and smart locks offers new opportunities for data annotation. Training machine learning systems on data from smart devices can lead to enhanced security features and smarter home solutions.
Conclusion: Unlocking Potential with Data Annotation
In conclusion, the impact of data annotation machine learning on businesses within the Home Services and Keys & Locksmiths industry is significant. As this technology continues to evolve and mature, it promises to transform how these businesses operate, making them more efficient, responsive, and customer-focused than ever.
By investing in quality data annotation processes, locksmiths can harness the power of machine learning to predict customer needs, enhance service offerings, and ultimately create a more secure environment for their clients. The future is bright for those willing to embrace the advancements in data annotation within the realm of machine learning.