Harnessing the Power of Labeling Tool Machine Learning for Business Growth
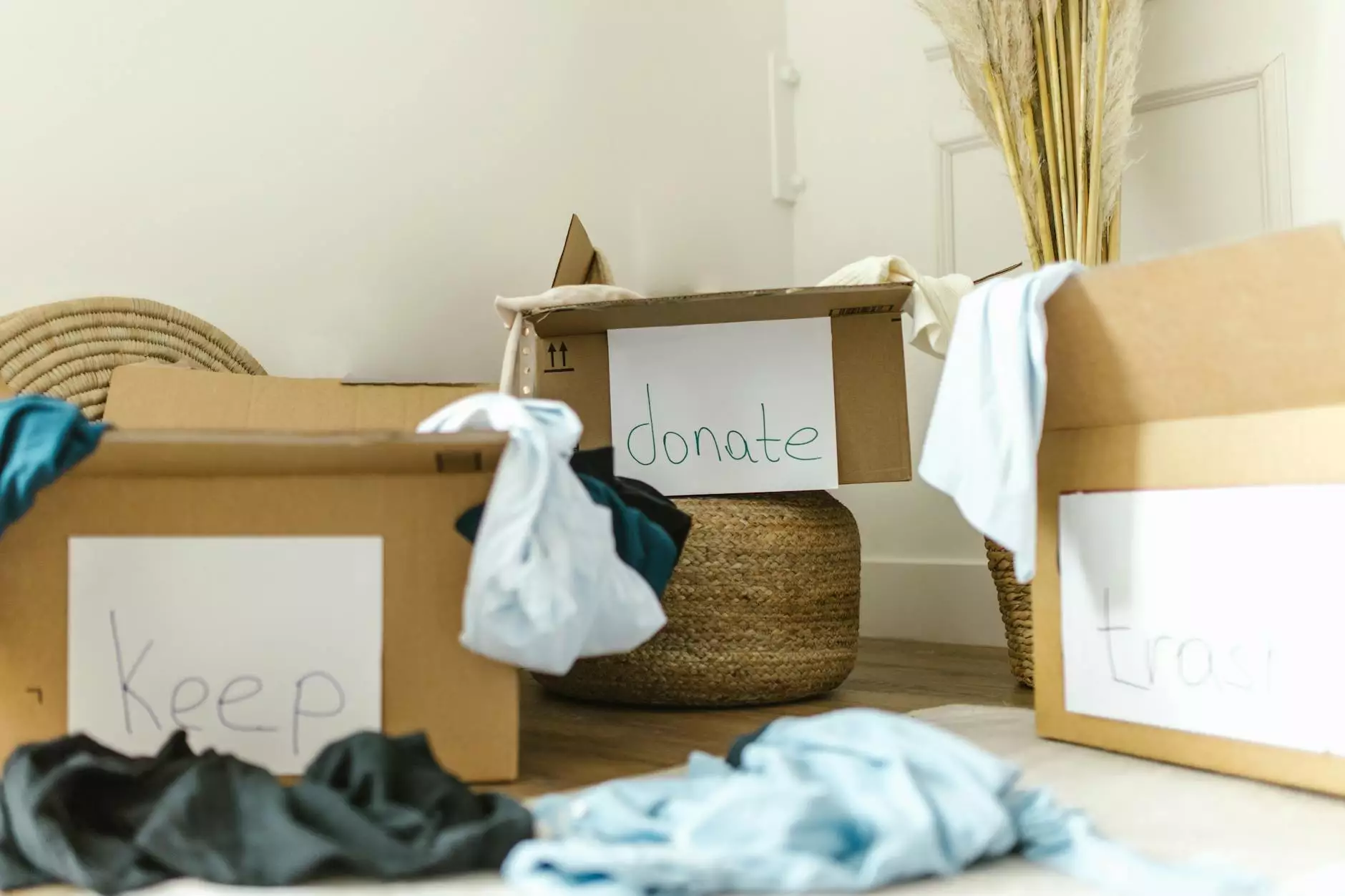
In today’s competitive landscape, businesses are increasingly turning to innovative solutions to boost efficiency and productivity. One such groundbreaking development is the labeling tool machine learning. By optimizing data annotation processes, companies can expedite their project timelines and improve the accuracy of their datasets. In this article, we will explore the nuances of labeling tools and how they can transform the way businesses approach data annotation.
Understanding the Fundamentals of Machine Learning and Data Annotation
Before diving into the specifics of labeling tools, it is important to understand the role of machine learning in data annotation. Machine learning, a subset of artificial intelligence, enables systems to learn from data and make predictions or decisions without being explicitly programmed. Data annotation involves the process of labeling data to train machine learning models. This crucial step ensures that algorithms can recognize patterns and improve their accuracy over time.
Why Data Annotation Matters
- Model Performance: Well-annotated data leads to better machine learning model performance, ensuring reliable predictions.
- Time Savings: Efficient data annotation can drastically reduce the time required for model training and deployment.
- Quality Output: High-quality labeled data minimizes errors and enhances the trustworthiness of results.
- Scalability: As businesses expand, so do their data needs. Scalable annotation solutions are essential for ongoing success.
Introducing Labeling Tool Machine Learning
The concept of a labeling tool machine learning integrates advanced algorithms and user-friendly interfaces to streamline the data annotation process. These tools enable users to label data efficiently, making it easier for machine learning models to learn and adapt. The automation of labeling tasks allows businesses to allocate their resources more effectively while reducing human error.
Types of Labeling Tools
There are various types of labeling tools available in the market, each catering to specific needs:
- Image Annotation Tools: Used for labeling images, detecting objects, and segmenting images for tasks like computer vision.
- Text Annotation Tools: Essential for labeling text, which might include sentiment analysis, named entity recognition, or text classification.
- Audio and Video Annotation Tools: Useful for transcribing audio data and labeling video frames for tasks like activity recognition.
Key Features of Effective Labeling Tools
When selecting a labeling tool for machine learning, businesses should look for the following features:
- User-Friendly Interface: The tool should be intuitive, allowing users to label data quickly and comfortably.
- Collaboration Capabilities: The ability to work collaboratively in real-time boosts productivity among team members.
- Integration with ML Frameworks: Seamless integration with popular machine learning frameworks enhances workflow efficiency.
- Automation Features: Incorporating machine learning for predicting labels can significantly speed up the annotation process.
- Reporting and Analytics: Tools should provide data insights to help teams assess the quality of their annotations.
The Benefits of Using Labeling Tool Machine Learning in Business
Implementing a labeling tool machine learning solution can yield numerous advantages for businesses looking to enhance their data annotation processes:
1. Increased Efficiency
Automated labeling can reduce the time spent on manual annotation, allowing teams to focus on high-value tasks. This efficiency directly translates to faster project completion and quicker time-to-market.
2. Enhanced Accuracy
Machine learning algorithms can refine label predictions over time, leading to more accurate data sets. The consistency offered by automated tools helps in minimizing human errors that can jeopardize model performance.
3. Cost-Effectiveness
By reducing the need for extensive manual work, businesses can save on labor costs associated with data labeling. Furthermore, the improved speed of model training allows for faster iterations and a more agile operation.
4. Scalability and Flexibility
Labeling tools can handle various types of data and adapt to specific business needs, making them an ideal solution for companies of all sizes. As data volumes grow, the right tool can scale up quickly without compromising performance.
5. Improved Collaboration
With robust collaboration features, teams can communicate and share insights during the labeling process. This synergistic approach diminishes knowledge silos and fosters a more cohesive team environment.
How to Choose the Right Labeling Tool for Your Business Needs
Selecting the right labeling tool for machine learning is an important decision that can impact your business operations. Here are some steps to guide you in making the right choice:
1. Assess Your Requirements
Identify the type of data you will be annotating (e.g., images, text, audio) and the volume of data that needs labeling. Understanding your specific needs will help narrow down your options.
2. Evaluate Usability
Choose a tool with an intuitive interface that your team can learn quickly. A steep learning curve can hinder productivity and lead to frustration.
3. Explore Automation Features
Look for automation capabilities that can speed up the labeling process. Tools that utilize machine learning for predictive labeling can significantly reduce manual workloads.
4. Check for Compatibility
Ensure that the tool integrates seamlessly with your existing machine learning frameworks and data management systems. Compatibility is key to an efficient workflow.
5. Consider Support and Resources
Opt for vendors that offer comprehensive support and resources. Having access to training materials, customer service, and a community forum can be beneficial for troubleshooting and learning.
Real-World Applications of Labeling Tool Machine Learning
Many industries are leveraging labeling tool machine learning to enhance their operations:
1. Healthcare
In the healthcare sector, labeling tools are pivotal for annotating medical images, which assists in diagnosing diseases and conditions. Accurate data labeling can lead to better patient outcomes and improved operational efficiencies.
2. Automotive
The automotive industry employs labeling tools for developing autonomous vehicles. Annotating data from various sensors is crucial for teaching vehicles to recognize objects and navigate their surroundings safely.
3. Retail
Retailers use labeling tools to analyze consumer behavior by annotating customer data and feedback. This information is critical for developing targeted marketing strategies and improving customer experiences.
4. Marketing and Advertising
In marketing, annotated data is essential for understanding audience sentiment and preferences. By labeling content effectively, businesses can craft more compelling messages that resonate with their target audiences.
Conclusion: Driving Business Success with Labeling Tool Machine Learning
Investing in a labeling tool machine learning solution can significantly enhance the efficiency and accuracy of data annotation processes within your business. By streamlining workflows, reducing costs, and enabling better collaboration, these tools empower organizations to harness the full potential of their data.
In a world where data reigns supreme, the ability to annotate data effectively will distinguish successful organizations from their competitors. Embrace the power of labeling tools today, and elevate your business to new heights of productivity and innovation.